The Need for Accurate Product Matching in Digital Retail
According to a Statista report, eCommerce will account for an estimated 21% of retail sales worldwide in 2022 up from 10% only 5 years ago. Forecasts indicate that by 2025, the online segment would make up close to 25% of total global retail sales. These numbers show the speed at which digital commerce is making strides in the global marketplace. In this evolved digital landscape, savvy shoppers have become more informed than ever and closely compare prices before making purchase decisions.
To convert these shoppers, brands and retailers need to stay one step ahead of their competitors by offering the best prices at all times. How can they do this at speed and scale without compromising on their margins? With advanced, automated, AI-driven product matching solutions that offer the highest accuracy levels, as, low quality, inaccurate data can have a direct impact on the bottom line and cost retailers, consumers. Intelligence Node’s award-winning product matching solution checks all the right boxes and offers unbeatable speed, accuracy, and scale in benchmarking against exact and similar competitor products – keeping its customers always ahead in the game.
Without 99% Product Matching Accuracy, You are Missing out on:
- Finding the most accurate matches for exact and similar products
- Benchmarking against competitors to offer the best prices
- Saving costs incurred due to inaccurate data and comparisons
- Improving product level margins, profitability, and brand loyalty
The following sections of the blog post will answer:
- Can I track similar and exact products sold by competitors?
- What all parameters can I track for benchmarking?
- Can I decide which attributes to consider for similar product matches?
- What if after finding a similar match, there is a better match available later?
Legacy Product Matching Challenges
Most legacy or manual product matching techniques use only one or two attributes to identify product matches. This method might not always work as often products sold online have missing information, don’t have UPC codes, have different nomenclature for the same products or have images or descriptions missing. This approach can also lead to a high error rate in finding the right matches alongside low accuracy levels. Such matching techniques have another problem attached to them – their inability to be fast, agile, and scalable. Many of these methods are manual tools that make use of spreadsheets to match products or they’re partially automated solutions that will not sustain when the business expands to include thousands of products with multiple competitors – leading to delays in finding matches, rising inaccuracies, and lost opportunities. Some of the common challenges that hamper accurate product matching are:
- No way of matching products without UPC codes
- Relying on only the name or main image to match products
- Absence of unique identifiers – mainly a problem across Fashion, Home, and Beauty products
- Slight variations in attributes along with incomplete specs
- Absence of standard nomenclature attributes leading to inaccurate or missed matches
- Inability to match private label products (unbranded) which are increasingly important
- Complications matching same product sold by multiple sellers under different brand names
Learn More : How Intelligence Node is Redefining Mobile App Scraping for Retail
Our Best-in-Class Product Matching with Unbeatable Accuracy
As a brand or a retailer, you want to ensure that any product matching you are doing is providing visibility into the comprehensive set of substitutes that influence your shoppers purchase choice. Today’s shoppers are making decisions based on a multitude of dynamic factors that can change in real-time. Leading brands and retailers digest this context and inform their go-to-market strategies accordingly.
Intelligence Node’s next generation product matching solution uses machine learning to match data and is powered by ‘Sherlock AI’ (proprietary AI technology) and patented ‘similarity engine’ which makes it highly intuitive, accurate, fast, and scalable. It uses a three pronged approach to product matching which enables it to match products despite missing UPC codes, inaccurate descriptions, varied nomenclature, and insufficient product descriptions:
- Key Product Attribute Comparison: We use product specific attributes for comparing products to ensure the match is valid.
- Image Comparison: We use image similarity (using CNN vector embeddings) to judge if a product-candidate pair looks visually similar.
- Text Comparison: We use two methods to achieve this – the first method is comparing word for word to identify an exact comparison and the second method is using a custom-tuned BERT for capturing semantic similarity between the product texts.
Using the above three-pronged approach ensures that all matches are vetted multiple times by looking at every aspect of the match. And in the few cases where the results derived by algorithms have a low probability, the matches are evaluated by data analysts to ensure a 99% accuracy level every time (it is written in our contractual SLAs). We further standardize the attribute nomenclature, identify miscategorized products, and ensure our matches are accurate from both ‘recall’ and ‘precision’ perspectives.
With Intelligence Node, retailers and brands can dominate the price war with our one-to-one identical matching for branded products and discover similar products for private labels with one-to-one similar matching. Moreover, Intelligence Node can tailor its matching algorithm to meet your internal criteria for tracking similar products in your category.
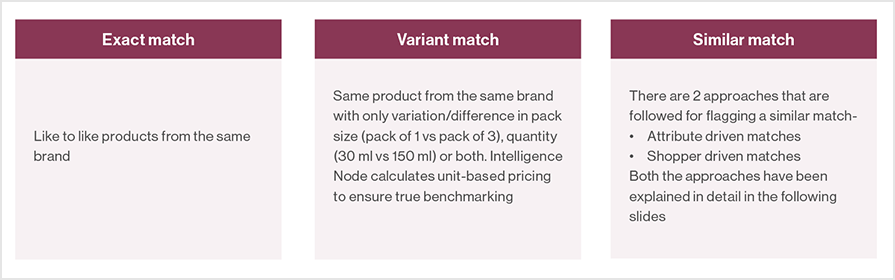
1. Exact Match – Like to Like Branded Matches
A simple and straightforward method to benchmark your prices and positioning against your competitors is to first identify the exact same products sold by your competitors and understand how they are pricing these products across the eCommerce universe. Our sophisticated crawlers scan the entire global eCommerce market to deliver all of the identical products across your competitive landscape so that you can craft a winning go-to-market strategy that converts shoppers at your point of purchase.
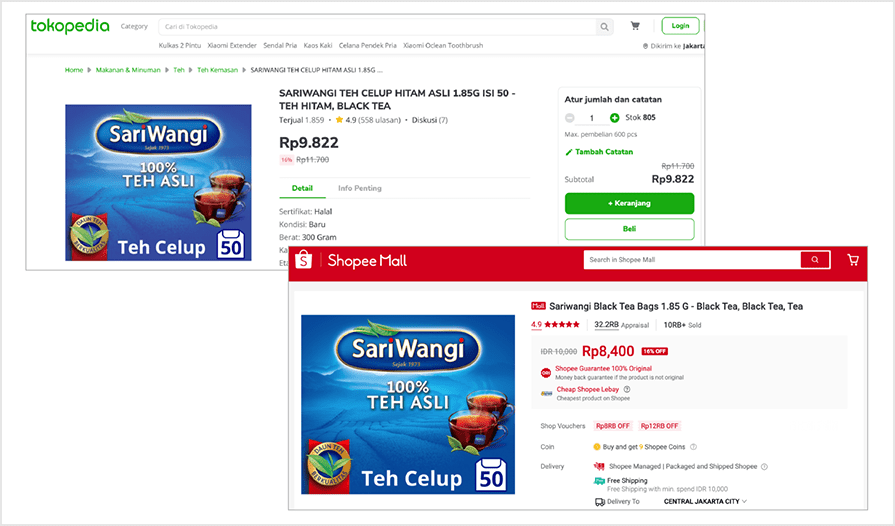
Both the products on Shopee and Tokopedia are exact same items from the same brand but Shopee’s prices are cheaper than Tokopedia.
Shopee | Tokopedia | |
Brand | Sariwangi | Sariwangi |
Product type | Tea bags | Tea bags |
Ingredient specification | Black tea | Black tea |
Volume | 1.85g | 1.85g |
Pack | 50 | 50 |
Container type | Box | Box |
Price | Rp 8,400 | Rp 9,822 |
2. Similar Match – Private Label Matches
There are many variables to finding similar matches to your products. For some a similar match might consist of certain attributes, for some, it might be the price range, and for others, it might be the visual proximity to a product. If you look at very few variables or follow a singular approach, chances are you miss out on close matches which can lead to incomplete data and affect the benchmarking accuracy in the long run.
Our AI-powered data listening capabilities continuously monitor websites around the world to help you find and compare with competitor products that are close matches and standardize attributes for accurate comparison. Additionally, we follow two different approaches to flagging similar matches to ensure no close match goes undetected.
Approach to flagging similar matches
Approach 1 – Based on product attributes
In this approach, a list of key attributes is defined for each category in collaboration with the client. Depending on whether or not the attributes match, the system will flag the competitor products as –
Attribute match
- All attributes are matched/within acceptable threshold
Rejected due to attribute mismatch
- One or more attribute is mismatched/not within acceptable threshold
Note:
- If requested by the user, this approach can also be dynamic in nature (i.e our algorithms will continuously keep searching for a better match)
- The user needs to define a relevant pool of candidates (i.e should the algorithm search for a match on the first 3 pages only or consider all products?)
Example:
We consider key product attributes that affect the buying decision to flag a similar match. Both the products on Walmart and BestBuy are 55” LED Smart TV. Since all attributes match, we have marked it as a Full attribute match.
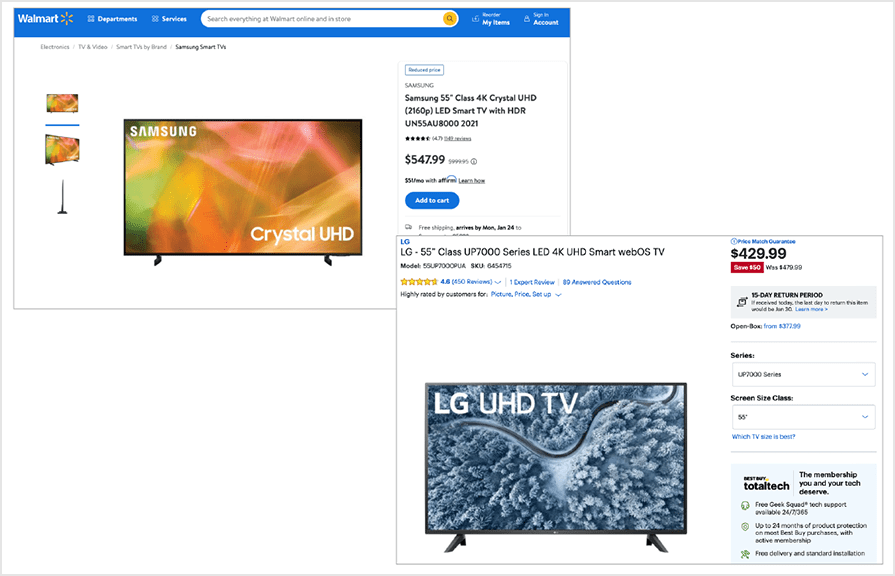
Walmart | Bestbuy | |
Brand | Samsung | LG |
Product category | TV | TV |
Sub type | UHD LED smart TV | UHD LED smart TV |
Screen | 55” | 55” |
Resolution | 4K | 4K |
Price | $547.99 | $429.99 |
Approach 2 – Shopper workflow based matching
In this approach, the objective is to identify the best match, from a set of products that the shopper might see, when they search for a specific product on the website.
- This approach can be best described as rule-based product matching where the algorithm creates various buckets based on the priorities defined by the user. It is important to note that the priorities can be customized based on the client’s business needs.
- Our algorithm uses search keywords or targeted terms that a shopper may use, to populate a pool of relevant products (or candidates). Eg – smoked chicken sausages, tomatoes etc
- Machine learning techniques such as visual similarity and context similarity are used to flag the best match available, from the available pool of candidates. Additional parameters might include price thresholds, etc based on the items in scope
- It should be noted that this approach may not be ideal for all product categories. For instance, a shopper who is looking to buy an electronic product would give more weightage to product attributes (and not visual and context similarity)
Note – If requested by the user, this approach can also be dynamic in nature (i.e our algorithms will continuously keep searching for a better match)
Example:
Today’s tech-savvy shoppers are price-sensitive. Let us consider an example of a Millennial shopper, Zoey. She always compares the price of a product across various websites before making a buying decision. Today, she wants to buy a box of frozen Applegate chicken sausages (as shown below)
At Walmart, it costs $4.54. In search of a better bargain, she decides to visit Amazon.com and looks for a similar product to compare the price difference!
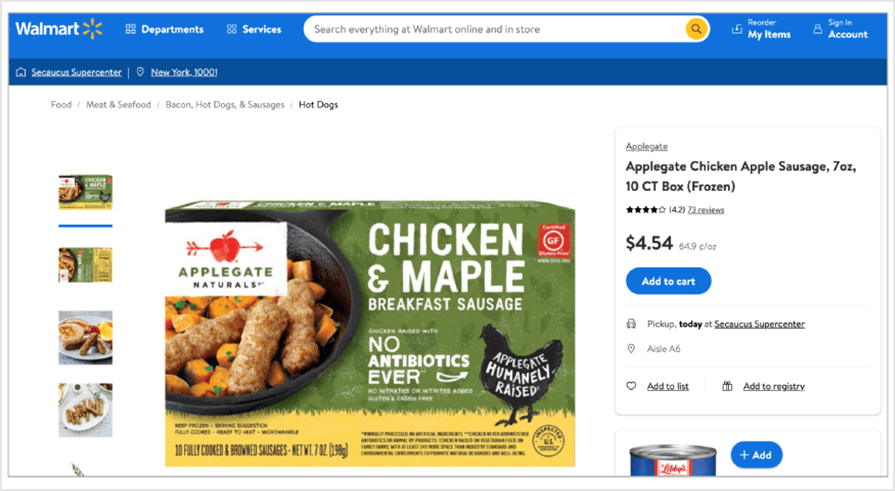
This is where Intelligence Node’s shopper workflow approach for finding similar matches comes in!
Just like Zoey, first, our algorithm looks for all relevant results related to the search keyword “Applegate chicken sausage”
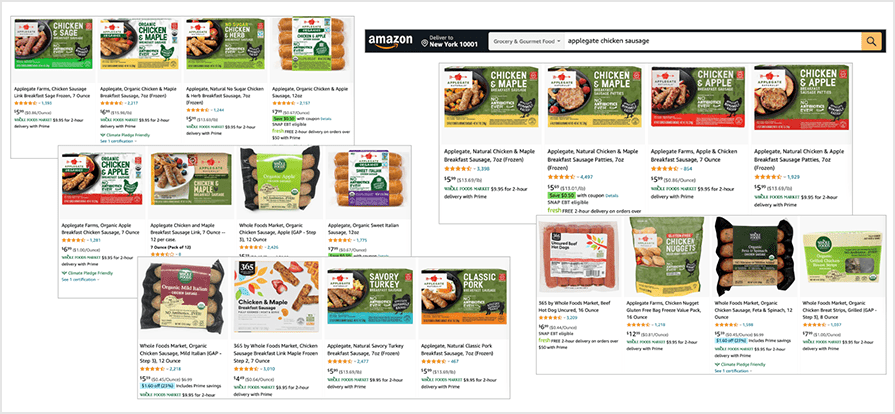
Priority 1 – Flagging products with the highest similarity score
Next, just like Zoey, the algorithm will try to identify the best possible match on Amazon for the product that was originally selected on Walmart.
Our AI algorithms generate image similarity score, context similarity score, and price threshold score to ultimately flag the product with the highest product similarity score.
In this case, the product with the highest product similarity score is flagged below –
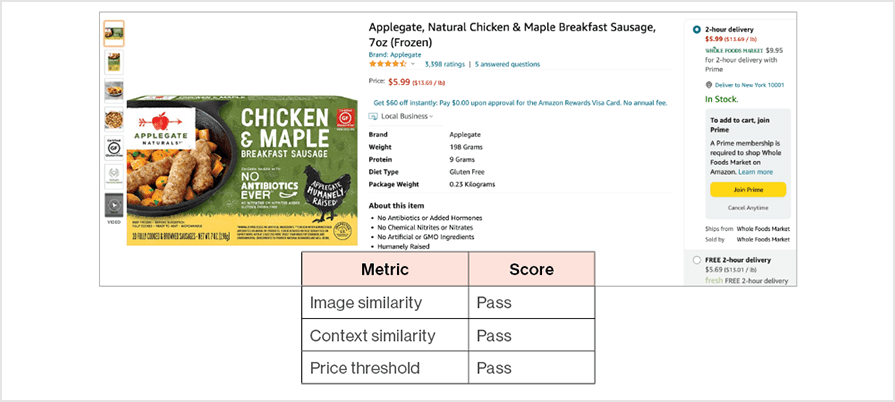
Priority 2 – Flagging products with high similarity score
Let us assume that the product on the previous slide is no longer available. In this scenario, just like Zoey, our algorithm will look for the next best match from the available pool of relevant candidates.
In this case, the next best match based on the product similarity score is flagged below –
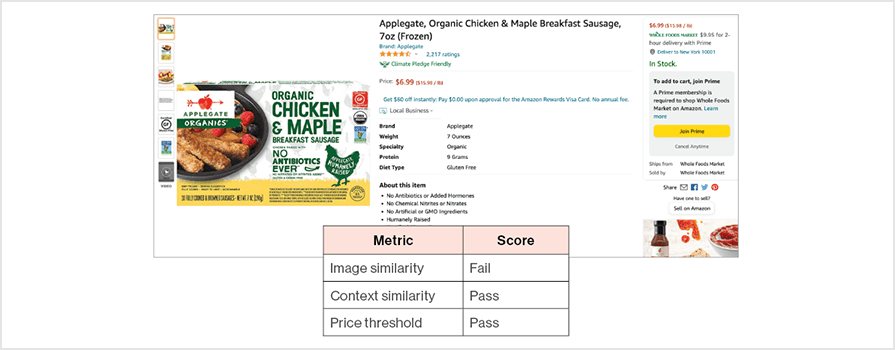
Priority 3 – Flagging products with moderate similarity score
Let us assume that the products on the previous slides are no longer available. In that case, just like Zoey, our algorithm will look for the next best match from the available pool of relevant candidates.
The next best match based on the product similarity score is flagged below –
Metric | Score |
Image similarity | Fail |
Context similarity | Fail |
Price threshold | Pass |
Priority 4 – Flagging products with low similarity score
Let us assume that the products on the previous slides are no longer available. In that case, just like Zoey, our algorithm will look for the next best match from the available pool of relevant candidates (although, the product similarity score would now reduce, thereby making it a less likely match).
The next best match (based on the product similarity score) would be –
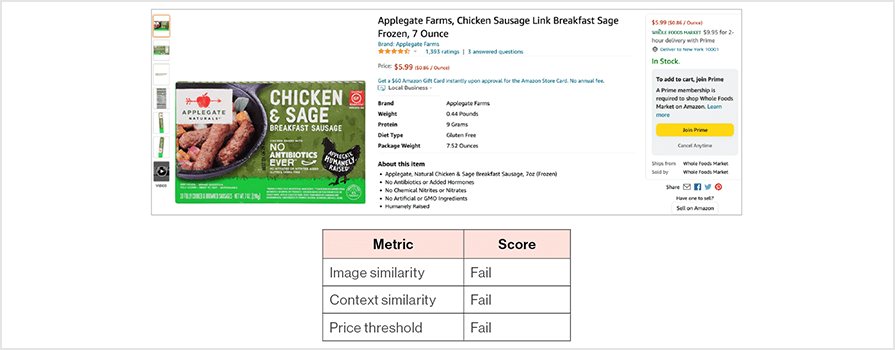
3. Variant Match
Shoppers aren’t just comparing the exact same products – they’re comparing your products across variations in size, color, and quantity, among other factors. Variant matching captures these factors to inform the optimization of your product offer, tipping the odds of winning the shopper in your favor.
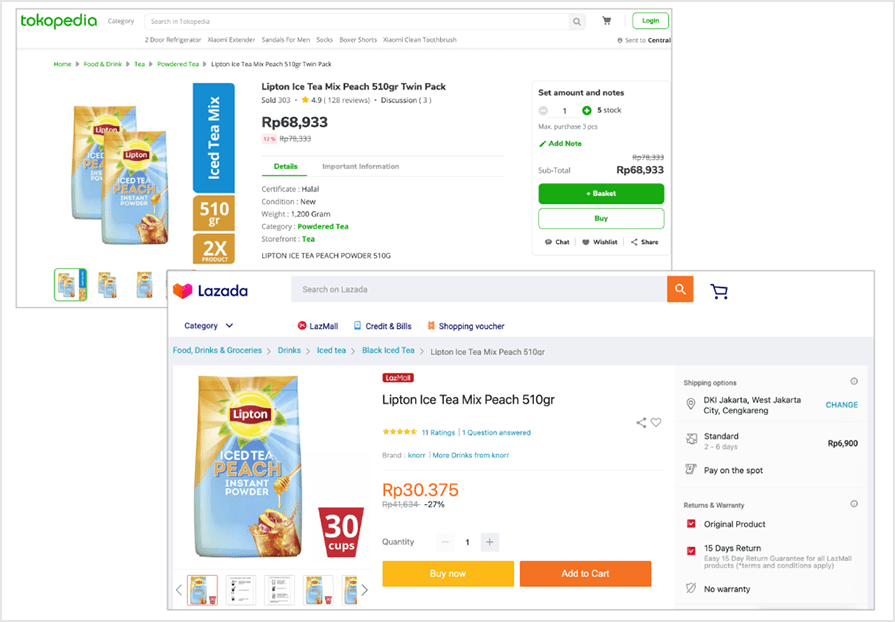
Both the products on Tokopedia and Lazada are peach flavored ice tea. However Tokopedia is available in pack of 2 whereas Lazada is in pack of 1. Since both products are identical in terms of brand,product type, etc and the only difference is pack size, we have marked it as Variant match.
We would be marking this as an Variant match in case Exact match product is not available.
Tokopedia | Lazada | |
Brand | Lipton | Lipton |
Product type | Ice tea | Ice tea |
Volume | 510g | 510g |
Pack | Pack of 2 | Pack of 1 |
Container type | Packet | Packet |
Ingredient specification | Tea powder | Tea powder |
Price | Rp 68,933 | Rp 30,375 |
Price per unit | Rp 67.58/g | Rp 59.55/g |
A Dynamic Approach to Product Matching
At Intelligence Node, we are always striving to enhance our product offerings by leveraging Artificial Intelligence and advanced, predictive analytics. We have done just that with our already powerful and highly accurate Product Matching solution. Instead of finding one time matches for our customers, we are now applying a dynamic approach to product matching where we continuously crawl competitor websites to find better, closer, and the most relevant matches for your products even in cases where there is already a similar or variant match identified in the first instance.
The below workflow breaks down the working of the dynamic pricing approach to Product Matching:
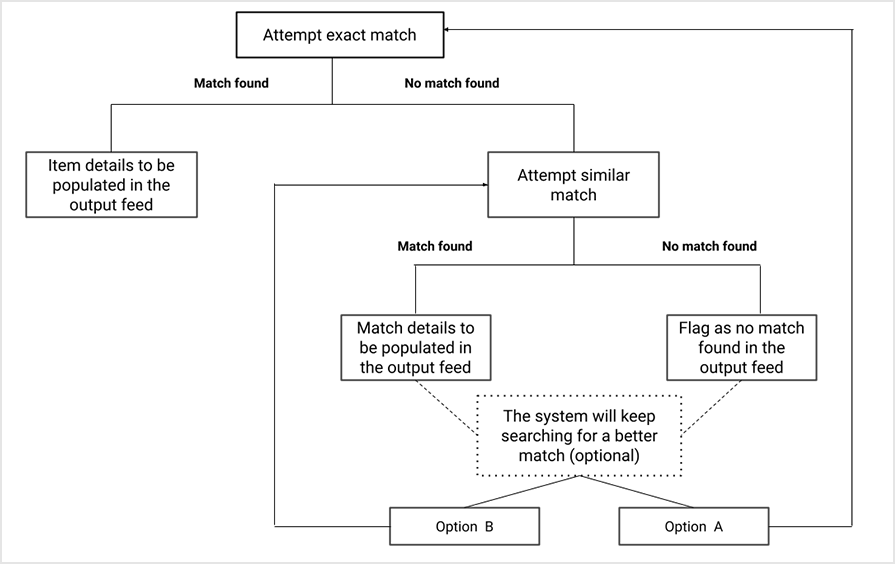
Example:
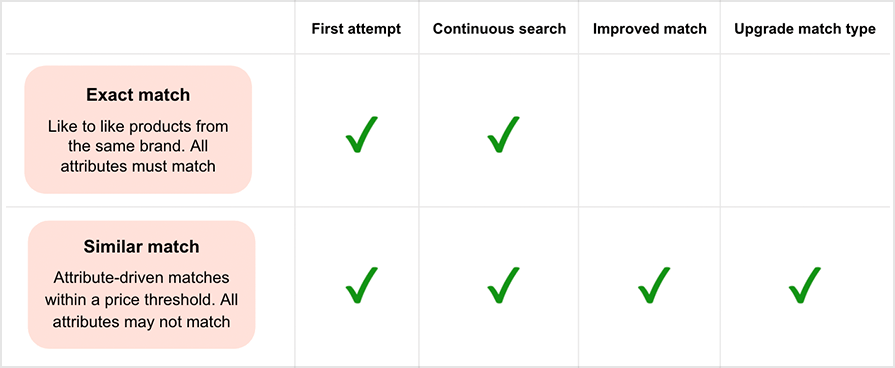
Day 1: Exact match not available: the system flagged a similar match
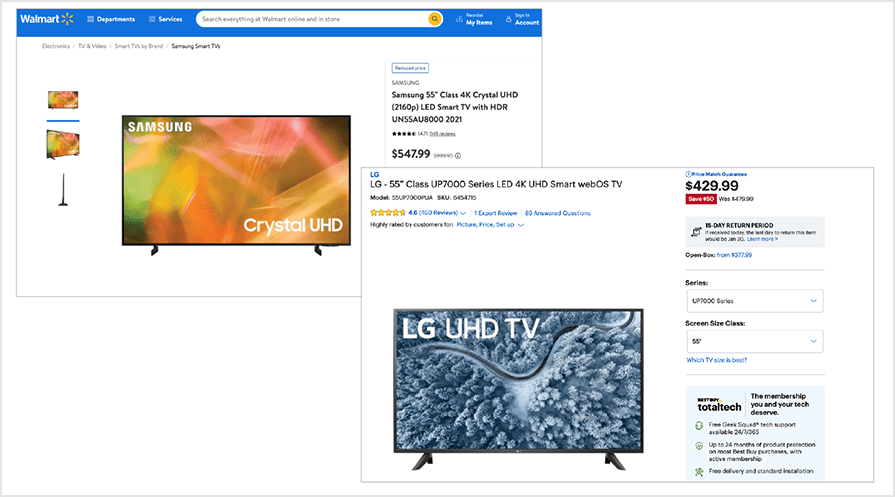
We consider key product attributes that affect buying decision to flag a similar match
Walmart | Bestbuy | |
Brand | Samsung | LG |
Product category | TV | TV |
Sub type | UHD LED smart TV | UHD LED smart TV |
Screen | 55” | 55” |
Resolution | 4K | 4K |
Price | $547.99 | $429.99 |
Day 12: A better similar match available, so the system replaced the previous match
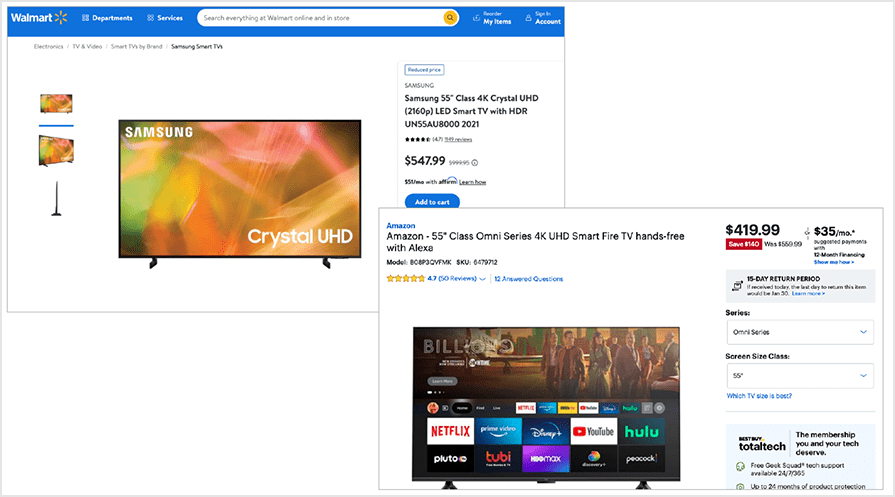
Day 23: An exact match available so the system replaced the previous similar match
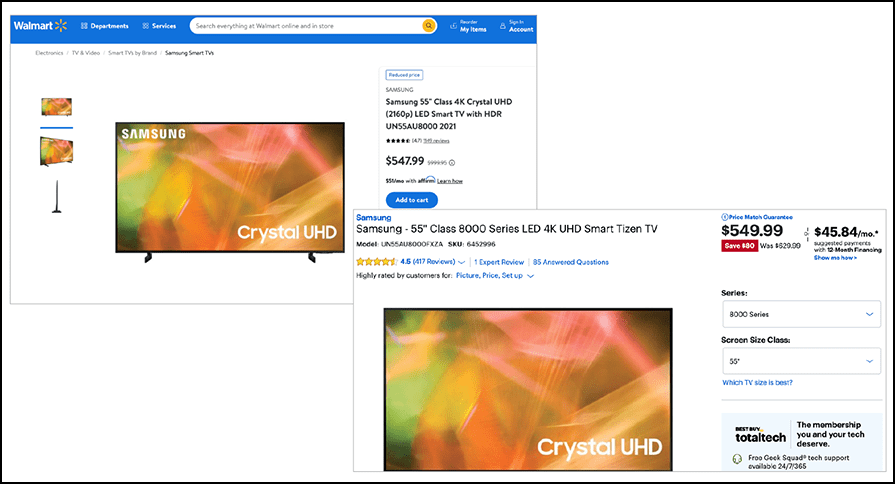
Final Word
As retailers and brands navigate the post-pandemic, channel-agnostic retail landscape, one this is undeniable and that is that data will largely drive decision-making across retail channels. Comparison shoppers will coax retailers and brands to offer competitive prices and assortments, making advance product matching tools for price optimization a common requirement across retail businesses. The sooner businesses realize the need for product matching solutions like Intelligence Node, that are fast, accurate, and automated, the more competitive advantage they will gain and have higher chances of future-proofing their businesses.
